Chapter 2 Context, Literature, and Rationale
2.1 Melodic Dictation
Melodic dictation is the process in which an individual hears a melody, retains it in memory, and then uses their knowledge of Western musical notation to recreate the mental image of the melody on paper in a limited time frame. For many, becoming proficient at this task is at the core of developing one’s aural skills (Karpinski 1990). For over a century, music pedagogues have valued melodic dictation,2 which is evident from the fact that most aural skills texts with content devoted to honing one’s listening skills have sections on melodic dictation (Karpinski 2000).
Yet despite this tradition and ubiquity, the rationales as to why it is important for students to learn this ability often comes from some sort of appeal to tradition or underwhelming anecdotal evidence. The argument tends to go that time spent learning to take melodic dictation results in increases in near transfer abilities after an individual acquires a certain degree of proficiency learning to take melodic dictation. Rationales given for why students should learn melodic dictation has even been described by Karpinski as being based on “comparatively vague aphorisms about mental relationships and intelligent listening” (Karpinski 1990, 192), thus leaving the evidence for the argument for learning to take melodic dictation not well supported.
Some researchers have taken a more skeptical stance and asserted that the rationale for why we teach melodic dictation deserves more critique. For example, Klonoski, in writing about aural skills education aptly questions: “What specific deficiency is revealed with an incorrect response in melodic dictation settings?” (Klonoski 2006). Earlier researchers like Potter, in their own publications, have noted that many musicians do not actually keep up with their melodic dictation abilities after their formal education ends (Potter 1990), but presumably go on to have successful and fulfilling musical lives. Additionally, suggesting that people who are unable to hear and then dictate music, thus presumably unable to think in music (Karpinski 2000), seems to forget many musical cultures that do not depend on written notation.
Despite this skepticism towards the topic, melodic dictation remains at the forefront of many aural skills classrooms. The act of becoming better at this skill may or may not lead to large increases in far transfer of ability, but when used as a pedagogical tool, the practice of learning to take melodic dictation intersects with current learning goals relevant to the core of undergraduate music training. While there has not been extensive research on melodic dictation research in recent years– in fact, Paney (2016) notes that since 2000, only four studies were published that directly examined melodic dictation– this skill set sits on the border between literature on music learning, melodic perception, memory, and music theory pedagogy. Understanding and modeling the processes underlying melodic dictation remains as an untapped watershed of knowledge for the field of music theory, music education, and music perception and is deserving of more attention.
In this chapter, I examine literature both directly and indirectly related to melodic dictation by first reviewing the prominent four-step model put forth by Karpinski in order to establish and describe melodic dictation. After describing his model, I then critique this model and put then forward a new taxonomy of parameters that presumably would contribute to an individual’s ability to take melodic dictation. Using this taxonomy, I then review relevant literature and assert that the next steps forward in understanding the underlying processes of melodic dictation by examining melodic dictation both experimentally and computationally. This research aims to continue to fill the long called for bridging between the fields of aural skills pedagogy and music cognition (Butler 1997; Karpinski 2000; Klonoski 2000).
2.1.1 Describing Melodic Dictation
The foundational pedagogical work on melodic dictation comes from the work of Karpinski. Summarized most recently in his Aural Skills Acquisition (Karpinski 2000)– though first presented in an earlier article (Karpinski 1990)– Karpinski proposes a four-step model of melodic dictation.
The four steps of Karpinski’s model include
- Hearing
- Short Term Melodic Memory
- Musical Understanding
- Notation
and occur as a looping process, which I have reproduced and depicted in Figure 2.1. Previous attempts to distill melodic dictation into a series of discrete steps have ranged from Michael Roger’s assertion of only needing two steps, to Ronald Thomas, who claimed as many as 15 steps, to similar models proposed by Colin Wright that model inner hearing as a five step model (Wright 2016; Karpinski 2000). Karpinski’s model is discussed extensively in both the original article (Karpinski 1990) and throughout the third chapter in his book (Karpinski 2000).
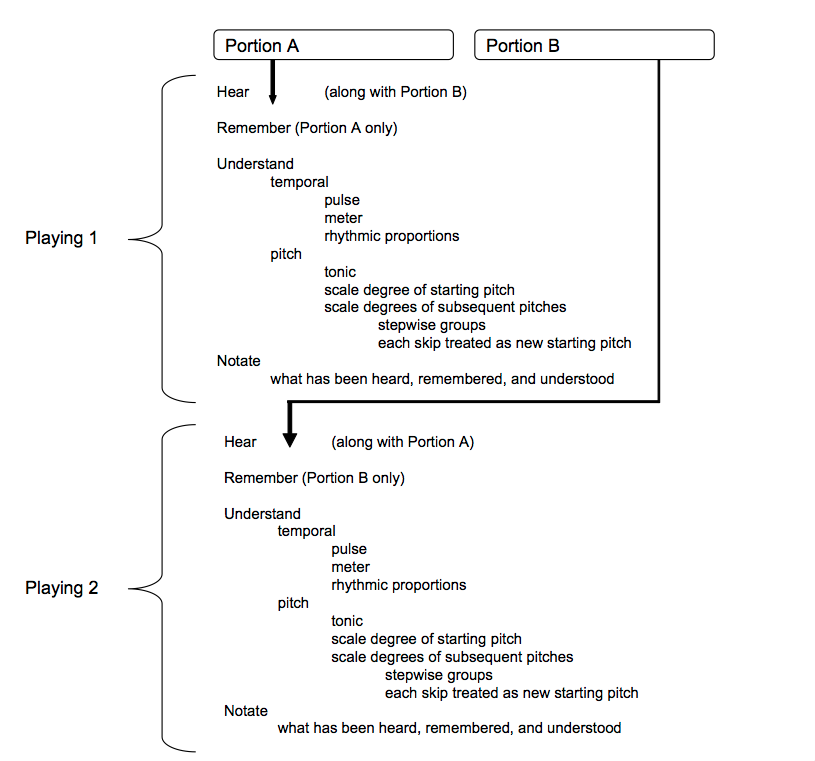
Figure 2.1: Karpinski Idealized Flowchart of Melodic Dictation
Karpinski’s hearing stage involves the initial perceptions of the sound at the psychoacoustical level and the listener’s attention to the incoming musical information. If the listener is not actively engaging in the task because of extrinsic factors such as “boredom, lack of discipline, test anxiety, attention deficit disorder, or any number of other causes.” (Karpinski 2000, 65) then any further processes in the model will be detrimentally affected. Karpinski notes that these types of interferences are normally “beyond the traditional jurisdiction of aural skills instruction” (Karpinski 2000, 65), but I will later argue that the concept of willful attention, when re-conceptualized as working memory, may actually play a larger role in the melodic dictation process as it is modeled here.
The short-term melodic memory stage in this process references the point in a melodic dictation where musical material is held in active memory. From Figure 2.1 and Karpinski’s writing on the model, this stage is not explicitly declared as any sort of active process akin to a phonological loop (Baddeley 2000) where active rehearsal might occur, but describes where in the sequential order melodic information is represented. Though Karpinski does not posit any sort of active process in the short term melodic memory stage, he does suggest there are two separate memory encoding mechanisms, one for contour, and one for pitch. He arrives at these two mechanisms by using both empirical qualitative interview evidence, as well as noting literature from music perception that supports this claim for contour (Dowling 1978; Dewitt and Crowder 1986) and literature suggesting that memory for melodic material is dependent on enculturation (Oura and Hatano 1988; Handel 1989; Dowling 1990). Since its publication in 2000, this area of research has expanded with other researchers also demonstrating the effects of musical enculturation via exposure (Eerola, Louhivuori, and Lebaka 2009; Stevens 2012; Pearce and Wiggins 2012; Pearce 2018).
In describing the short term melodic memory stage, Karpinski also details two processes that he believes to be necessary for this part of melodic dictation: extractive listening and chunking. Noting that there is a capacity limit to the perception of musical material by citing Miller (1956), Karpinski explains how each strategy might be incorporated. Extractive listening is the process in which someone dictating the melody will selectively remember only a small part of the melody in order to lessen the load on memory. Chunking is the process in which smaller musical elements can be fused together in order to expand how much information can be actively held and manipulated in memory. The concept of chunking is very helpful as a pedagogical tool, but as detailed below, is complicated to formalize.
After musical material is extracted and then represented in memory, the next step in the process is musical understanding. At this point in the dictation, the individual taking the dictation needs to mentalize the extracted musical material that is represented in memory and then use their music theoretic knowledge in order to comprehend any sort of hierarchical relationships between notes, common rhythmic groupings, or any sorts of tonal functions. This is the point in the process where solmization of either or both pitch and rhythm, and musical material might be understood in terms of relative pitch. While Karpinski reserves his discussion of solmization for the musical understanding phase, it is worth questioning if it is possible to disassociate relative pitch relations that would be ‘understood’ in this phase from the qualia of the tones themselves (Arthur 2018). For Karpinski, the quicker what is represented in musical memory can be understood, the quicker it can then be translated at the final step of notation.
Notation, the final step of the dictation loop, requires that the individual taking the notation have sufficient knowledge of Western musical notation so that they are able to translate their musical understanding into written notation. This last step is ripe for errors and has proved problematic for researchers attempting to study dictation (Taylor and Pembrook 1983; Klonoski 2006). It is also worth highlighting that it is difficult to notate musical material if the individual who is dictating does not have the requisite musical category and knowledge for the sounds that are actively represented in memory. Lack of this knowledge will limit an individual’s ability to translate what is in their short term melodic memory into notation, even if accurately represented in memory.
Nearer the conclusion of the chapter, Karpinski notes that other factors like tempo, the length and number of playings, and the duration between playings also play a role in determining how an individual will perform on a melodic dictation. While this framework can help illuminate this cognitive process and help pedagogues understand how to best help their students, presumably there are many more factors that contribute to this process. The model as it stands is not detailed enough for explanatory purposes and lacks in two areas that would need to be expanded if this model were to be explored experimentally and computationally.
First, having a single model for melodic dictation assumes that all individuals are likely to engage in this sequential ordering of events. This could in fact be the case3, but there is research from music perception (Goldman, Jackson, and Sajda 2018) and other areas of memory psychology such as work on expert chess players (Lane and Chang 2018) that suggests that as individuals gain more expertise in a specific domain, their processing and categorization of information changes. Additionally, different individuals will most likely have different experiences dictating melodies based on their own past listening experience, an area that Karpinski refers to when citing literature on musical enculturation based on statistical exposure. The model does not have any flexibility in terms of individual differences.
Second, the model presumes the same sequence of events for every melody. As a general heuristic for communicating the process, this model serves as an excellent didactic tool. When this model is applied to more diverse repertoire, this same set of strategies performed in this order might prove to be inefficient. For example, on page 103 of his text, Karpinski suggests that two listenings should be adequate for a listener with few to no chunking skills to be able to dictate a melody of twelve to twenty notes. This process might generalize to many tonal melodies, but presumably different strategies in recognition would be involved in dictating the two melodies of equal length shown in Figures 2.2 and 2.3. If asked to dictate 2.2, long term memory processes might begin to play a role much sooner during this task. If asked to dictate 2.3, establishing a tonal center to act as a perceptual scaffolding for relative pitch relationships might prove to be more difficult. Presumably different people with different levels of abilities will perform differently on different melodies.
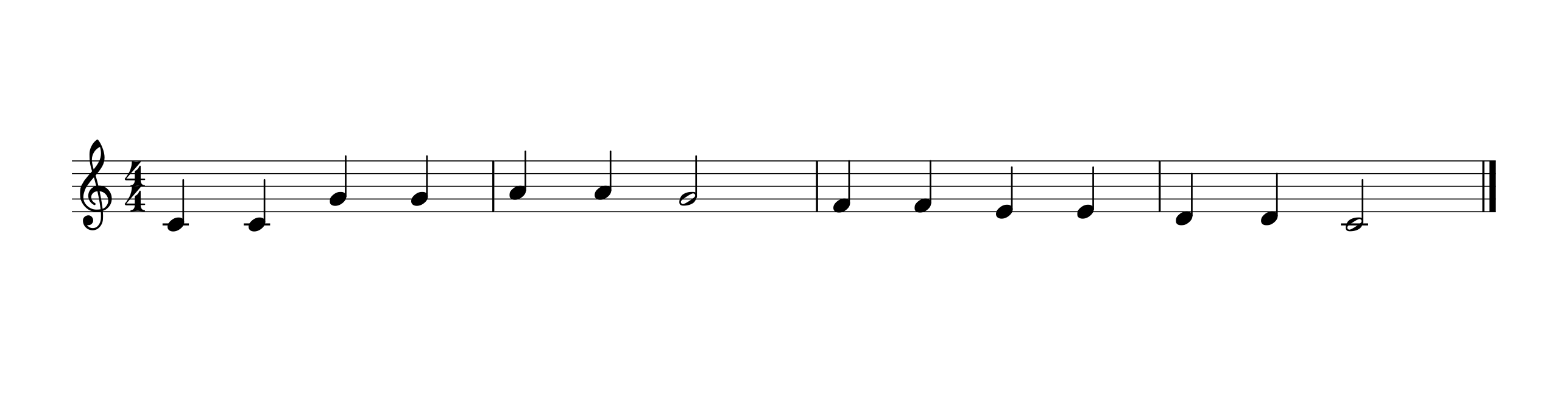
Figure 2.2: Tonal Melody
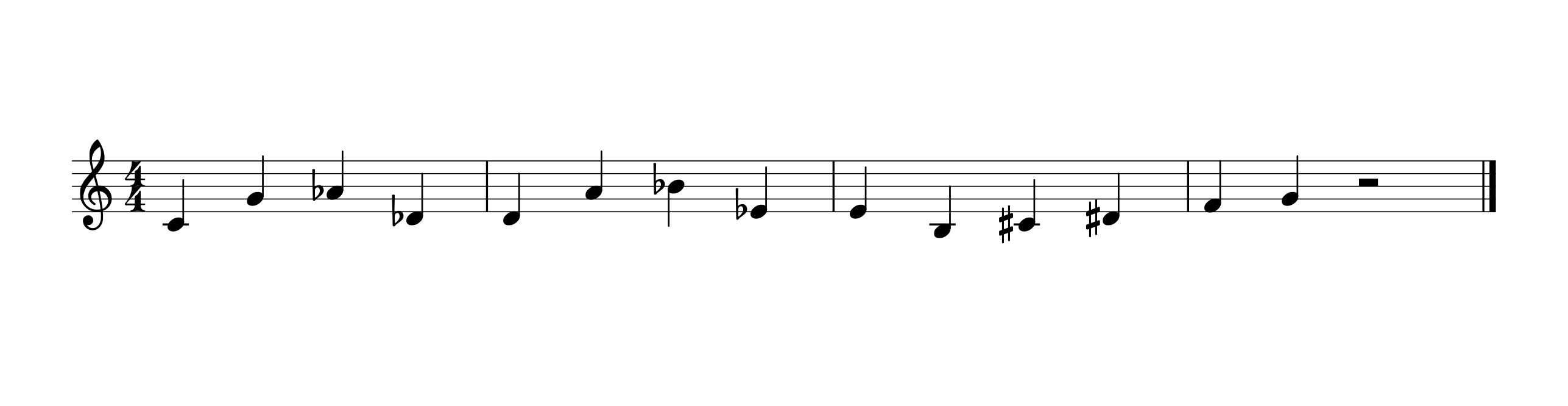
Figure 2.3: Atonal Melody
This agnosticism for both variability for melodic and individual differences serves as a stepping off point for this study. In order to have a more complete understanding of melodic dictation, a model should be able to accommodate the exhaustive differences at both the individual and musical levels. Additionally, the model should be able to be operationalized so that it can be explored in both experimental and computational settings. Explicitly stating variables thought to contribute the underlying processes of melodic dictation will give aural skills pedagogues a more exact framework for discussing melodic dictation. In turn, this will enable a more complete understanding of melodic perception and subsequently allow for better teaching practices in aural skills classrooms.
2.1.2 Taxonomizing
At this point, it is worth stepping back and noting that the sheer amount of variables at play here is cumbersome and haphazard. In order to better understand and organize factors thought to contribute to this process, it would be advantageous to taxonomize the multitude of features thought to contribute to melodic dictation. In doing this, it will allow for a clearer picture of what factors might contribute and what literatures to explore in order to learn more about them.
The taxonomy that I propose appears in Figure 2.4 and bifurcates the possible factors thought to affect an individual’s ability to take melodic dictation create both individual parameters and musical parameters. These categories are recursively partitioned into cognitive and environmental parameters as well as structural and experimental factors respectively. Below I expand on what these categories entail, then explore each in-depth.
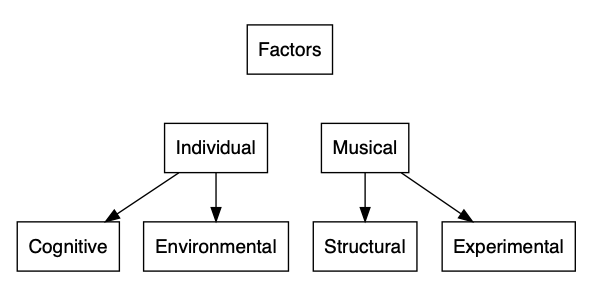
Figure 2.4: Taxonomy of Factors Contributing to Aural Skills
The individual parameters are split broadly into cognitive factors and environmental factors. Factors in the cognitive domain are assumed to be relatively consistent over time. Factors in the environmental domain are subject to change via training and exposure. These categories are not deterministic, nor exclusive, and almost inevitably interact with one another.
For example, it would be possible to imagine an individual with higher cognitive ability, the opportunity to have a high degree of training early on in their musical career, and personality traits that lead them to enjoy engaging with a task like melodic dictation. This individual’s melodic dictation abilities might be markedly different than someone with lower cognitive abilities, no opportunity for individualized training, and not have a general inclination to even take music lessons. This variability at the individual level might then lead to differences in their ability to take melodic dictation.
Complementing the individual differences, there would also be differences at the musical level which in turn divides into two categories. On one hand exists the structural aspects of the melody itself. These are aspects of the melody that would remain invariant when written down on musical notation that can only capture pitch changes over time. Parameters in this category would include features generated by the interval structure of the pitches over time that allow the melody to be perceived as categorically distinct from other melodies. These structural features are then complimented by the experimental features which are emergent properties of the structural relation of the pitches over time based on performance practice choices. Examples of these parameters would include, key, tempo, note density, tonalness, timbral qualities, and the amount of times a melody is played during a melodic dictation. Again, this division is not an exhaustive, categorical divide. One could imagine exceptions to these rules where a major mode melody played on the piano is transformed to the minor mode, ornamented, and then played with extensive rubato by a trumpet. This new melody might be experienced as a phenomenologically distinct, yet similar experience. This division of structural and experimental features is similar to Leonard Meyer’s primary and secondary musical features (Meyer 1956).
Given all of these parameters that could contribute to the melodic dictation process, the remainder of this chapter will explore literature using this taxonomy as a guide. The chapter concludes with a reflection on operationalizing each of these factors and problems that can arise in modeling. These are important to note since from an empirical standpoint, both the task as well as the process of melodic dictation as depicted by Karpinski resemble a process that could be operationalized as both an experiment, as well as a computational model.
2.2 Individual Factors
2.2.1 Cognitive
Research from cognitive psychology suggests that individuals differ in their perceptual and cognitive abilities in ways that are both stable throughout a lifetime and are not easily influenced by short term training. When investigated on a large scale, these abilities– such as general intelligence or working memory capacity– predict a wealth of human behavior on a large scale ranging from longevity, annual income, ability to deal with stressful life events, and even the onset of Alzheimer’s disease (Ritchie 2015; Unsworth et al. 2005). Given the strength and generality of these predictors, it is worth investigating the extent that these abilities might contribute when investigating any modeling of melodic dictation, since melodic dictation depends on perceptual abilities. It is important to understand the degree to which these cognitive factors might influence aural skills abilities in order to ensure that the types of assessments that are given in music schools validly measure abilities that individuals have the ability to improve. If it is the case that much of the variance in a student’s aural skills academic performance can be attributed to something the student has little control over, this would call for a serious upheaval of the current model of aural skills teaching and assessment.
Recently there has been interest in work exploring how cognitive factors are related to abilities in music school4. This interest is probably best explained by the fact that educators are picking up on the fact that cognitive abilities are powerful predictors and need to be understood since they inevitably will play a role in pedagogical settings. Before diving into a discussion regarding differences in cognitive ability, I should note that ideas regarding differences in cognitive ability have been negatively received and for good reasons. Research in individual differences in cognitive ability can and has been taken advantage to further specious ideologies, but often arguments that assert meaningful differences in cognitive abilities between groups are founded on statistical misunderstandings and have been addressed in other literature (Gould 1996). These differences cannot be brushed aside because it is very difficult to maintain a scientific commitment to the theory of evolution (Darwin 1859) and not expect variation in all aspects of human behavior, with cognition falling under that umbrella.
2.2.1.1 General Intelligence
Attempting to measure and quantify aspects of cognition date back over a century. Even before concepts of intelligence were posited by Charles Spearman via his conception of g (Spearman 1904), scientists were interested in establishing links between latent constructs they presumed to exist in the real world– yet were impossible to measure directly like intelligence– and physical manifestations that could be measured such as body morphology (Gould 1996). While scholars like Gould have documented and critiqued much of the history of early psychometrics5, central to this study are two important schools of thought on intelligence testing commonly discussed in the current literature.
The first ideology originates from Cyril Burt and Charles Spearman who, in developing the statistical tool of factor analysis, posited that a construct of general intelligence exists as a part of human cognition and can be quantified. Burt and Spearman claimed that a general intelligence factor existed in human cognition from evidence they put forward developing a battery of cognitive tests whose performance on one subtest could often reliably predict performance on another. This phenomena of multiple related tests predicting each other’s performance is a manifestation referred to as the positive manifold. Spearman and Burt asserted that an individual’s ability to solve problems without contextual background information could be understood as general intelligence or g (Spearman 1904).
Broadly speaking, the second ideology here stems from work by Alfred Binet, who instead of conceptualizing intelligence as a monolithic whole, partitioned intelligence into what today has become understood to be defined as differences in general crystallized intelligence or ( Gc ) and general fluid intelligence ( Gf ). General crystallized intelligence is the ability to solve problems given prior contextual information; General fluid intelligence is the ability to solve problems in novel contexts (Cattell 1971; J Horn 1994) . Comparing Gf and Gc to g, the cognitive psychology literature has noted that g often shares a statistically equivalent relationship to an idea conceptualized as general fluid intelligence (Matzke, Dolan, and Molenaar 2010). These conceptions of intelligence and cognitive ability also differ from more current theories that synthesize these previous areas of research (Kovacs and Conway 2016) using models that do not require taking an ontological stance of entity realism (Borsboom, Mellenbergh, and van Heerden 2003).
Even though both of these constructs are powerful predictors on a large scale and do predict variables such as educational success, income, and even life expectancy (Ritchie 2015) when confounding variables like socioeconomic status are accounted for, conceptualizing cognitive abilities in terms of only a handful of latent constructs still does not fully explain the diversity of human cognition. Regardless of their origin, neglecting the predictive power of these variables in pedagogical settings would be a methodological oversight in attempting to explain variance in performance.
2.2.1.2 Working Memory Capacity
In addition to concepts of intelligence, be it Gf or Gc, the working memory capacity literature directly relates to work on melodic dictation. Working memory is one of the most investigated concepts in the cognitive psychology literature. According to Nelson Cowan, the term working memory generally refers to
the relatively small amount of information that one can hold in mind, attend to, or, technically speaking, maintain in a rapidly accessible state at one time. The term working is meant to indicate that mental work requires the use of such information. (p.1) (Cowan 2005)
The term, like most concepts in science, does not have an exact definition, nor does it have a definitive method of measurement. While there is no universally recognized first use of the term, researchers began to postulate that there was some sort of system that mediated incoming sensory information from the external world with information in long term storage using modular models of memory in the mid-twentieth century. Summarized in Cowan (2005), one of the first modal models of memory was proposed by Broadbent (1958) and later expanded by Atkinson and Shiffrin (1968). As seen in Figure 2.5 taken from Cowan (2005), both models here posit incoming information that is then put into some sort of limited capacity store. These modal models were then expanded on by Baddeley and Hitch (Baddeley and Hitch 1974) in their 1974 chapter with the name Working Memory, where they proposed a system with an central executive module that was able to carry out active maintenance and rehearsal of information that could be stored in either a phonological store for sounds or a visual sketchpad for images.
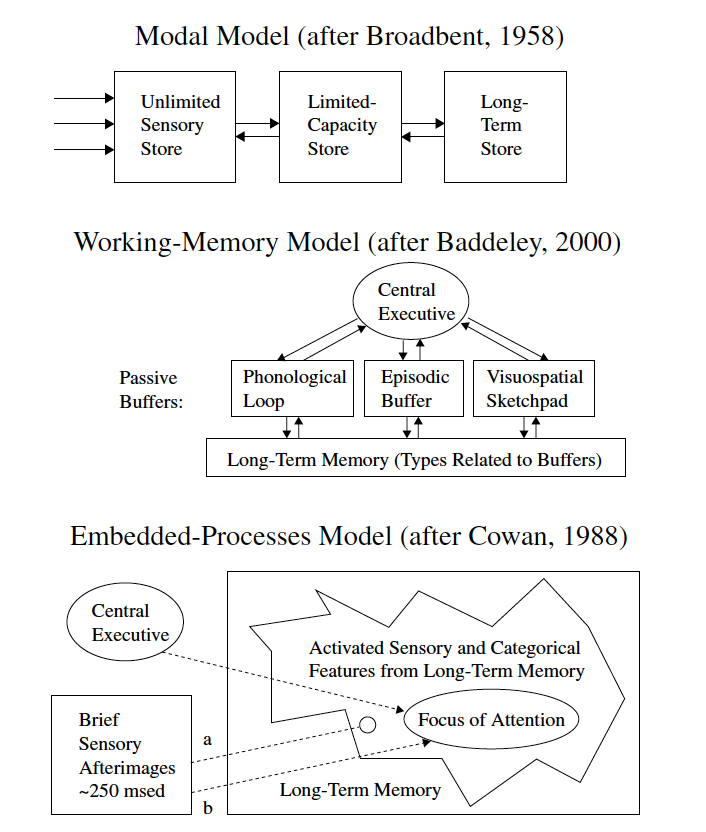
Figure 2.5: Schematics of Models of Working Memory taken from Cowan, 2005
Later revisions of their model also incorporated an episodic buffer (Baddeley 2000) where the modules were explicitly depicted as being able to interface with long term memory in the rehearsal processes. The model has even been expanded upon by other researchers throughout its lifetime. The most relevant to this study is by Berz (1995), who postulated the addition of a musical rehearsal loop to the already established phonological loop and visual spatial sketchpad. While Berz is most likely correct in asserting that the nature of storing and processing musical information is different to that of words or pictures and there has been experimental evidence to suggest this (Williamson, Baddeley, and Hitch 2010) that has been interpreted in favor of multiple loops (Wöllner and Halpern 2016), the idea of multiple loops introduces the theoretical problem of determining how and why incoming sensory information is partitioned into their respective loops. Additionally, models that assert some sort of central executive component to attend to materials held in a sensory buffer also face the infinite regress homunculus problem. Stated more clearly, if the central executive system is what attends to information in the sensory buffers, what attends to the central executive?
In addressing the problem of explicitly stating which rehearsal loops do and do not exist, Nelson Cowan proposed a separate model (Cowan 1988, 2005), dubbed the Embedded Process Model, which does not claim the existence of any domain specific module (e.g. positing a phonological loop, visual spatial sketchpad) but is rather based on an exhaustive model that did away with the problem of asserting specific buffers for specific types of information.
In Cowan’s own words comparing his model from that of Baddeley and Hitch:
The aim was to see if the description of the processing structure could be exhaustive, even if not complete, in detail. By analogy, consider two descriptions of a house that has not been explored completely. Perhaps it has only been examined from the outside. Baddeley’s (1986) approach to modeling can be compared with hypothesizing that there is a kitchen, a bathroom, two equal-size square bedrooms, and a living room. This is not a bad guess, but it does not rule out the possibility that there actually are extra bedrooms or bathroom, that the bedroom space is apportioned into two rooms very different in size, or that other rooms exist in the house. Cowan’s (1988) approach, on the other hand, can be compared with hypothesizing that the house includes food preparation quarters, sleeping quarters, bathroom/toilet quarters, and other living quarters. It is meant to be exhaustive in that nothing was left out, even though it is noncommittal on the details of some of the rooms. (p.42) (Cowan 2005)
The system is depicted in the bottom tier of Figure 2.5, and conceptualizes the limited amount of information that is readily available as being in the focus of attention. In this model, activated sensory and categorical features of the focus of attention are thus readily accessible. Moving further from the locus of attention is long term memory, whose content can be activated using the Central Executive to access non-immediately available information. The central executive system in this case acts as a spotlight on what is represented in long term memory, rather than a module used to direct attention to specific sensory information. This change in definition does not completely escape the homunculus problem, but does change the central executive’s role in the memory process. In contrast to the modular approaches, Cowan’s framework does not require researchers to specify exactly how and where incoming information is being stored. This makes it advantageous for studying complex stimuli such as music and melodies. Using this definition of working memory would require collapsing the first two steps of the Karpinski model of melodic dictation into one step. In this case, attention is the window of active memory.
In addition to having multiple frameworks for studying working memory capacity, there is also the problem of limits to the working memory system, often referred to as the working memory capacity. Most popularized by Miller in his famous (George A Miller 1956) speech turned article, Miller suggests out of jest that the number 7 might be worthy of investigating in terms of how many items can be remembered, which has been used as a point of reference for many researchers since then. It is worth nothing that Miller has gone on record as noting that using 7 (plus or minus 2) was a rhetorical device inteneded to string together his speech and not a claim to pursue the number seven as the limit for memory (Miller 1989). Nevertheless, while the number seven is most likely a red herring, it did inspire a large amount of research on capacity limits. In the decades since, the number 7 has been reduced to about 4 (Cowan 2010) and research around capacity limits has been investigated using a variety of novel tasks, most notably the complex span task (Unsworth et al. 2005, 2009). When complex span tasks are used as a measure of working memory capacity, they tend to be both valid and reliable psychometric tools that are stable across a lifetime (Unsworth et al. 2005).
2.2.1.3 Working Memory and Melodic Dictation
Clearly an individual’s ability to take in sensory information, maintain it in memory and actively carry out other tasks are almost identical to tasks of working memory capacity. Before venturing onward and further discussing the importance of this striking parallel, a few clear distinctions between methods used to study working memory and melodic dictation need to be made explicit. While these two tasks resemble each other, a few key differences exist that researchers must note.
Tasks investigating working memory capacity differ from melodic dictation tasks in a few key ways. The first is that musical information is always sequential: a melodic dictation task would never require the student to recall the pitches back in scrambled orders. Serial order recall is an important characteristic in the scoring and analyzing of working memory tasks (Conway et al. 2005), but musical tones do not appear in random order and are normally in discernible chunks as discussed by Karpinski (Karpinski 2000). The use of chunks is pervasive in any literature on working memory and is often used as a heuristic to help explain why information is grouped together. Of the problems with chunking, most are related to music and are related to music and thus melodic dictation. Below I review the problems with chunking noted by Cowan (2005), and how each confound would manifest itself in music related research.
- Chunks may have a hierarchical organization. Tonal music has historically been understood to be hierarchical (Krumhansl 2001; Meyer 1956; Schenker 1935) with the study of memory for tones being confounded by some pitches being understood by their relation to structurally more stable tones.
- The working memory load may be reduced as working memory shifts between levels in hierarchy. If an individual understands a chunk to be something such as a major triad, the load on working memory would be less since that information could be understood as a singular chunk.
- Chunks may be the endpoints of a continuum of associations. Given tonal music’s sequential and statistical properties, two tones might be able to be loosely associated given a context that would make the tones fall between being identified as two separate tones and one distinct chunk.
- Chunks may include asymmetrical information. More tonal possibilities are possible from a stable note like tonic or dominant, whereas in a tonal context, a raised scale degree #\(\hat{4}\) when understood in a functional context would be taken as having stricter transitional probabilities (#\(\hat{4} \rightarrow \hat{5}\)).
- There may be a complex network of associations. If a set of pitches sounds like a similar set of pitches from long term memory, the incoming information cannot be understood as being separate units of working memory.
- Chunks may increase in size rapidly over time. Three tones that are seemingly unrelated when played sequentially. E4, G5, C5 might enter sensory perception as three distinct tones, but then be fused together if understood as one chunk– a first inversion major triad.
- Information in working memory may benefit from rapid storage in long term memory. Given the amount of patterns that an individual learns and can understand, as soon as sensory information is fused, it could be encoded in long term memory. This is especially true if there is a salient feature in the incoming melodic information such as the immediate recognition of a mode or cadence.
The points by Cowan are important to acknowledge in that it is not possible to directly lift work and paradigms from working memory capacity to work in music perception. That said, the enormous amount of theoretical frameworks put forward by the working memory literature when understood in conjunction with theories in music psychology, such as implicit statistical learning (Saffran et al. 1999), can provide for new, fruitful theories. Past researchers have noted the strength and predictive abilities from the working memory capacity literature as aiding research in music perception. In ending his article positing a musical memory loop to be annexed to the Baddley and Hitch modular model of working memory, Berz (1995) captures the power of this concept in the last sentence of his article and warns:
Individual differences portrayed in some music aptitude tests may [sic] represent not talent or musical intelligence but ability, reflecting differences in working memory capacity. p. 362
Berz’s assertion has not been exhaustively tested since first published in 1995, but the subject of music, memory, and cognitive abilities has been the focus of research for both both psychologists and musicologists alike.
2.2.1.4 Working Memory Capacity and Music
Of the papers in the music science literature that specifically investigate working memory, each uses different measures, but all tend to converge on two general findings. The first is that there are some sort of enhanced memory capabilities in individuals with musical training. The second is that working memory capacity, however it is measured, often plays a significant role in musical tasks. Evidence for the first point appears most convincingly in a recent meta analyses by Talamini and colleagues (Talamini et al. 2017), who demonstrated via three separate meta-analyses, that musicians outperform their non-musical counterparts on tasks dealing with long-term memory, short-term memory, as well as working memory. The authors also noted that the effects were the strongest in working memory tasks where the stimuli were tonal, which again suggests an advantage of exposure and understanding of the hierarchical organization of musical materials. In this meta-analyses and that from others investigating music and cognitive ability, it is important to be reminded that the direction of causality from these studies still cannot be determined using these theoretical and statistical methodologies. While it might seem that musical training tends to lead to these increases, it is also possible that higher functioning individuals will self select into musical activities. Even if there is no selection bias in engaging with musical activity, it also remains a possibility that of the people who do engage with musical activity, the higher functioning individuals will be less likely to quit over a lifetime.
In terms of musical performance abilities, working memory capacity has also been shown to be a significant predictor. Kopiez and Lee suggested that working memory capacity should contribute to sight reading tasks based on research where they found measures of working memory capacity, as measured by a matrix span task, to be significantly correlated with many of their measures hypothesized to be related to sight reading ability in pianists at lower difficulty grading (Kopiez and Lee 2006, 2008).
Following up on this work on sight reading, Meinz and Hambrick (Meinz and Hambrick 2010) found working memory capacity, as measured by an operation span task, a reading span task, rotation span task, and a matrix span task was able to predict a small amount of variance \(R^2=.07 (0.07)\) above and beyond that of deliberate practice alone \(R^2=.45 (.44)\) in a sight-reading task. More recently, two studies looking at specific sub-groups of musicians have shown working memory capacity to significantly contribute to models of performance on musical tasks related to novel stimuli. Wöllner and Halpern (2016) found that although they did not observe differences between pianists and conductors in measures of working memory capacity as measured via a set of span tasks, conductors showed superior performance in their attention flexibility. Continuing this line of research Nichols, Wöllner, and Halpern (2018), used the same battery of working memory tasks and found that jazz musicians excelled over their classically trained counterparts in a task which required them to hear notes and reproduce them on the piano. The authors also noted that of their working memory battery, based on standard operation span methods (Engle 2002), that the auditory dictation condition scored surprisingly low, and further research might consider work on dictation abilities. Additionally, Colley, Keller, and Halpern (2017) found working memory capacity, as measured by a backwards digit span and operation span, to be successful predictors in a tapping task requiring sensory motor prediction abilities. As mentioned above, each of these tasks where working memory was a significant predictor of performance occurred where the task involved active engagement with novel musical material.
The growing evidence in this field suggests that having larger working memory capacity to be greatest in both musically trained people, and that these effects are the most pronouced dealing with novel, tonal information. Since all three of these factors are related to melodic dictation, it would seem sensible to continue to include these measures in tasks of musical perception and continue Berz’s assertion that research in music perception could inadvertently be picking up on individual differences in working memory abilities.
2.2.1.5 Intelligence and Music
As discussed above, the idea of IQ or intelligence has a long and complex history. When used as a predictor in statistical models, it often serves to predict traits that society values like longevity and general income, so given its ability to predict in more domain general settings, surveying literature where applicable to musical activity warrants attention. Below I use the term intelligence as a catch all term to avoid the historical context of IQ, and to specify, where available, which measure was used. Before surveying the literature, it is also worth noting that research on music and intelligence is not as developed as some of the larger studies that look at intelligence, which creates problems for both establishing causal directionality, as well as controlling for other factors like self-theories of ability, socioeconomic status, and personality (Müllensiefen et al. 2015).
As reviewed in Schellenberg (2017), both children and adults who engage in musical activity tend to score higher on general measures of intelligence than their non-musical peers (Gibson, Folley, and Park 2009; Hille et al. 2011; Schellenberg 2011; Schellenberg and Mankarious 2012) with the duration of training sharing a relationship with the extent of the increases in IQ (Corrigall and Schellenberg 2015; Degé, Kubicek, and Schwarzer 2011; Schellenberg 2006). Though many of these studies are correlational, they have also have made attempts to control for confounding variables like socio-economic status and parental involvement in out of school activities (Corrigall and Trainor 2011; Degé, Kubicek, and Schwarzer 2011; Schellenberg 2011; Schellenberg and Mankarious 2012). Schellenberg notes the problem of smaller sample sizes in his review (Corrigall and Trainor 2011; Parbery-Clark et al. 2011; Strait et al. 2012) in that studies that are typically smaller do not reach statistical significance. Schellenberg also references evidence that when professional musicians are matched with non-musicians from the general population, there do not seem to be these associations (Schellenberg 2006). His review suggests the current state of the literature might be interpreted as higher functioning kids tend to gravitate towards music lessons, then subsequently persist with the lessons. Additionally, Schellenberg remains skeptical of any sort of causal factors regarding increases in IQ (Francois et al. 2013; Moreno et al. 2009) noting methodological problems like how short exposure times were in studies claiming increases in effects, or researchers not holding pre-existing cognitive abilities constant (Mehr et al. 2013). Continued work by Swaminithan, Schellenberg, and Khalil continue to support evidence for this selection bias in training resulting in higher cognitive abilities among musicians (Swaminathan, Schellenberg, and Khalil 2017). Under my taxonomy, the individual, cognitive traits such as general intelligence and working memory are presumed to be stable over a life time. I now turn to individual traits that are more malleable, those dubbed the Environmental traits.
2.2.2 Environmental
Standing in contrast to factors that individuals do not have a much control over such as the size of their working memory capacity or factors related to their general fluid intelligence, most of the factors music pedagogues believe contribute to one’s ability to take melodic dictation are related to what I have put forward as environmental factors. In fact, one of the tacit assumptions of any formal education revolves around the belief that with deliberate and attentive practice, an individual is able to move from novice status to some level of expertise in their chosen domain. The idea that time invested results in beneficial returns is probably best formalized by work produced by Ericsson, Krampe, and Tesch-Romer (1993) that suggests that performance at more elite levels results from deliberate practice.
As noted in studies above, such as Meinz and Hambrick (2010), deliberate practice is able to explain variance in task performance, but other research suggests more variables are at play. Detterman and Ruthsatz (1999) propose that three factors, general intelligence, domain specific ability, and practice are the cornerstones of developing expertise in music. The first of their three factors is not normally believed to be malleable, while the former two are presumed to be plastic. This reasoning has been explored by researchers such as Ruthsatz et al. (2008), who investigated these assertions and provided empirical evidence to support this notion using a hierarchical multiple regression modelling and concluded that each of these variables does in fact contribute significantly to the target variable of musical performance. Other researchers have since commented on these expertise models like Mosing et al. (2014) who have asserted that a genetic component, rather than those listed above best explain variance on musical ability.
One major problem interpreting literature like the studies mentioned above is the general lack of agreement on what constitutes musical behaviors. At a very high level, many of the aforementioned studies take a parochial view of what it means to engage in musical activity, a problem which is only exacerbated by not having uniform psychometric measurements (Baker et al. 2018; Talamini et al. 2017). Interpreting this data then becomes difficult as what it means to be proficient at a musical task is culturally dependent. Investigating musical talent as if it were a universal is a problem well documented in both the ethnomusicological and music education literature (Blacking 2000; Murphy 1999).
2.2.2.1 Aural Training
In addition to individuals differing in their general musical abilities– however they are defined– individuals also differ in their abilities at the level of their aural skills. The same problems that arise in operationalizing musicianship are apparent in defining aural skills. Reviewing the literature, I operationalize aural skills to encompass the many skills often taught in music school, not restricting those skills to any particular sets of exercises. Some researchers like Chenttee (Chenette 2019) have taken stricter definitions attempting to state only skills that engage working memory capacity as those that are truly aural, but this operationalization would limit this review’s scope.
Though not as heavily researched in the past few decades (Furby 2016), there has been specific research looking at modeling how individuals perform in aural skills examinations. Harrison, Asmus, and Serpe (1994) examined the effect of aural skills training on undergraduate students by creating a latent variable model investigating musical aptitude, academic ability, musical expertise, and motivation to study music in a sample of 142 undergraduate students and claimed to be able to explain 73% of the variance in aural skills abilities using the variables measured. Work from Colin Wright’s dissertation incorporated a mixed methods approach investigating correlations between aural ability and their degree success as well as interviewing university students regarding the importance of aural skills education. In his work he found a general positive correlation between aural ability and measures of degree success.
While results are still mixed regarding how best to measure and assess this ability, the near ubiquity of aural skills education has resulted in many investigations of how people might improve their ability. As noted in Furby (2016), researchers in the past have suggested a variety of techniques for improving abilities in melodic dictation by isolating rhythm and melody (Banton 1995; Bland 1984; Root 1931), listening attentively to the melody before writing (Banton 1995), recognizing patterns (Banton 1995; Bland 1984; Root 1931) and silently vocalizing while dictating (Klonoski 2006). Interpreting a clear best path forward from these studies again remains difficult due to the sheer amount of variables at play.
Often described as the other side of the same coin of melodic dictation, sight singing is an area of music pedagogy research that has received some attention, yet probably not the extent deserved given its prevalence in school of music curricula. Recently, Fournier et al. (2017) cataloged and categorized fourteen different strategies that students used when learning to sight read. The authors organized their fourteen categories into four larger main categories and suggested that aural skills pedagogues should employ their framework in their aural skills pedagogy in order to better communicate effective sight singing strategies.
Similar to commentaries in literature on melodic dictation, Fournier et al. (2017) also note a line of research that has documented that university students are often unprepared to sight-read single lines of music (Asmus 2004; Thompson 2003) even though it is, like dictation, thought of as a means for deeper musical understanding (Karpinski 2000; Rogers 2004). Fournier et al. (2017) also documented that sight-reading has been an active area of research because of the often reported relationship. Performance on sight reading often predicts links between academic success in sight-singing and predictors such as entrance tests (Harrison 1987), academic ability, and musical experience (Harrison, Asmus, and Serpe 1994).
Taken as a whole, the research tends to suggest that learning to be a fluid and competent sight reader helps musicians hone their skills by bootstrapping other musical skills since those needed for sight-reading touch on many of the skills used in musical performance such as pattern matching and listening for small changes in intonation. While the above literature suggests there are empirical grounds to consider these individual factors in predicting how well an individual will do in melodic dictation, these factors will invariably interact with the other half of the taxonomy: the musical parameters.
2.3 Musical Factors
Transitioning to the other half of the taxonomy on Figure 2.4, the other main source of variance in any study investigating melodic dictation is the effect of the melody itself. I find it safe to assume that not all melodies are equally difficult to dictate and assert that variance in the difficulty of the melody can be partitioned between both structural and experimental aspects of a melody. As noted above, there is not a strict delineation between these two categories since once could imagine manipulations in experimental parameters in order to result in a phenomenologically different experience of melody. Questions of transformations of melodies and musical similarity have been addressed in other research (Cambouropoulos 2009; Wiggins 2007) and are beyond the scope of this study.
2.3.1 “The Notes”
The assumption that a musical score is able to provide insights towards meaningful understanding is a core tenant of music theory and analysis. Throughout the 20th Century, music theorists have almost exclusively relied on musical scores as their central point of reference in their work. According to Clarke (Clarke 2005), this structuralist approach to music lays at the foundation of many academic discussions, possibly stemming from latent assumptions regarding absolutism in music. General interest in structure has been a dominant part of the discourse as evidenced from the extensive lines of thought emanating from Heinrich Schenker (Schenker 1935; Salzer and Mannes 1982; Schachter 2006; Schenker, Siegel, and Schachter 1990) and variations on linking what one might colloquially refer to as “the notes” to some sort of musical meaning is the lifeblood of music theory. While issues surrounding “the notes” as they pertain to discourse have been central to large debates within the musicological community (Agawu 2004; Kerman 1986), tethering “the notes” to explain phenomenological listening experiences in music received much of its theoretical framework from the work of Leonard Meyer and assertions he put forward in Emotion and Meaning in Music (Meyer 1956). In his text, Meyer posits that much of a listener’s experience in music can be understood by considering a listener’s expectations which are generated from the statistical properties of the music.
Research in Meyer’s tradition inspired work investigating the perception of melodic structures via the work of Eugene Narmour (Narmour 1990, 1992), Glenn Schellenberg (Schellenberg 1997), Elizabeth Hellmuth Margulis (Margulis 2005), and David Huron (Huron 2006). Meyer has also been the cited source of inspiration for recent, successful implementations of models of human auditory cognition like that of Marcus Pearce’s Information Dynamics of Music (Pearce 2005, 2018), which derives from information theoretic models of musical perception put forward by Ian Witten and Darrel Conklin (Conklin and Witten 1995).
Though even prior to Meyer and Schenker, one of the earliest researchers that sought to make an explicit link between “the notes” and perception comes from outside the dominant academic musicological discourse. The first study to examine the link between what might be understood as “the notes” and explicit memory was Otto Ortmann in 1933 (Ortmann 1933). Ortmann used a series of twenty five-note melodies in order to examine the effects of repetition, pitch direction, conjunct-disjunct motion (contour), interval size, order, and chord structure, all of which he deemed to be the determinants of an individual’s ability to recall melodic material. Though Ortmann did not use any statistical methods to model his data, he did assert that each of his determinants contributed to an individual’s ability to recall musical material. This work was extended by Taylor and Pembrook (1983) who additionally incorporated using musical skill as a predictor and subsequently found evidence that these factors contributed to individual dictation abilities in a sample of 122 undergraduate students.
What Ortmann referred to as determinants are structural aspects of the melody that can then be mapped to some aspect of perception. While Ortmann used the term determinants, for the rest of this study I instead adopt the term feature which better reflects current terminology used to talk about these aspects of a melody. Given Ortmann’s design of using isorhythmic five tone sequences, his detriments– or features– under my taxonomy from Figure 2.4 would generally include only structural aspects. Were Ortmann to have increased the tempo of the tones he presented, change the timbre of their instrumentation, or maybe provide participants more attempts to give their responses, he would have then been adjusting what I am referring to as the experimental parameters. In the section below, I first explore literature that set out to understand certain structural aspects of the musical side of my taxonomy, then begin to introduce studies that incorporate more parameters.
As with the above problems listed in attempting to measure latent psychological constructs, similar problems also arise in operationalizing many of the musical constructs in the experiments from above. Unlike individual features, since musical scores can be digitized, attempting to create more objective measurements for musical features is more straightforward than that of measuring latent psychological variables. One way to accomplish this is to use symbolic features of the melodies themselves as a variable to be measured. Unfortunately, much of the work from computational musicology such as David Huron’s Humdrum toolbox (Huron 1994) or Michael Cutberth’s Music21 (Cuthbert and Ariza 2010) pre-dates some of the earlier experimental work I will discuss below, but as these computations are more straightforward than considering larger experimental designs, I begin with them here. While I reserve a longer discussion on the histories of computational musicology for Chapter Four, relevant to this review are the additional ways it is now possible to abstract features from symbolic melodies beyond what was capable in studies such as Ortmann (1933) and Taylor and Pembrook (1983).
2.3.2 Abstracted Features
An abstracted symbolic features of a melody are emergent properties of the melody that result from performing a calculation on the melody when digitized into discrete, computer readable tokens. Abstracted symbolic features of melodies can largely be conceptualized as being static or dynamic. Static features of melodies are obtained by summarizing some aspect of the melody as if it were to be experienced in suspended animation. For example, a static feature of a melody might be the melody’s range as calculated by the number of half steps from the lowest to the highest note or the number of notes in a melody. Using static features helps quantify something that might be intuitive about a melody or piece of encoded music. For example, David Huron’s contour class used in a study investigating melodic arches (Huron 1996) using the Essen Folksong Collection (Schaffrath 1995) can only be understood as a feature of the melody itself once the melody has been sounded and recalled would be a static feature of a melody. Other examples include a melody’s global note density, normalized pairwise variability index (Grabe 2002), and a melody’s tonalness as calculated by one of the various key profile algorithms (Krumhansl 2001; Albrecht and Shanahan 2013). These measures are useful when describing melodies and are predictive of various behavioral phenomena as detailed below, but at this point it has not been well established to what degree these summary features can be directly and reliably mapped to aspects of human behavior.
The quintessential and most comprehensive toolbox example of this is Daniel Müllensiefen’s Feature ANalysis Technology Accessing STatistics (In a Corpus) or FANTASTIC toolbox (Mullensiefen 2009). FANTASTIC is software that is capable summarizing musical material at for monophonic melodies. In addition to computing 38 features such as contour variation, tonalnesss, note density, note length, and measures inspired by computational linguistics (Manning and Schütze 1999) FANTASTIC is also able to calculate m-types (melodic-rhythmic motives) that are based on the frequency distributions of melodic segments in musical corpora.
Work using the FANTASTIC toolbox has been successful in predicting court case decisions (Müllensiefen and Pendzich 2009), predicting chart successes of songs on the Beatles’ Revolver (Kopiez and Mullensiefen 2011), memory for old and new melodies in signal detection experiments (Müllensiefen and Halpern 2014), memory for ear worms (Jakubowski et al. 2017; Williamson and Müllensiefen 2012), memorability of pop music hook (Balen, Burgoyne, and Bountouridis 2015). In experimental studies, FANTASTIC has also been used to determine item difficulty (Baker and Müllensiefen 2017; Harrison, Musil, and Müllensiefen 2016) and has even been the basis of the development of a computer assisted platform for studying memory for melodies (Rainsford, Palmer, and Paine 2018).
In addition to using summary based features on melodies, it is also possible to model the perception of musical materials by using a dynamic approach that is dependent on the unfolding of musical material. First explored by Witten and Conklin (Conklin and Witten 1995), and then implemented as a dynamic model of human auditory cognition in his doctoral dissertation, Marcus Pearce’s Information Dynamics Of Melody (IDyOM) models musical expectancy using information theoretic concepts (Shannon 1948). The model takes an unsupervised machine learning approach and calculates the information content for musical events based on multiple pre-specified viewpoints (Pearce 2005). As a model, IDyOM has has been successful in modeling human responses to expectation, melodic boundary formation, and even measurements of cultural proximity (Pearce and Wiggins 2012; Pearce 2018). The domain general application of IDyOM has given credence to Meyer’s assertion that the enculturation of musical styles stems from statistical exposure to musical genres and is somewhat reflective of the cognitive processes used in musical perception. IDyOM has also been recently extended to look at expectation in polyphonic work (Sauve 2017) and expectations of harmony (Harrison and Pearce 2018).
The advantage of using a dynamic approach, as opposed to a static one, is that a dynamic approach theoretically reflects real-time perception of music with the structural characteristics of the music mapping on to real human behavior since expectancy values are calculated for every musical event. While employing this type of model does allow for calculations to be made for every musical event in question, the assumption also brings into question whether a computer model is able to calculate each musical event and be reflective of human cognition, and does that mean that the human perceptual system is also making on-the-fly probability calculations during perception? This problem is worthy of mention as it currently exists in literature on implicit statistical learning (Perruchet and Pacton 2006) and some researchers have put forward similarity based models that have claimed to explain processes attributed to statistical learning, but do not depend on the statistical learning mechanism (Jamieson and Mewhort 2009).
2.3.2.1 Ecological Experiments
While the field of computational musicology has built models for quantifying these perceptual aspects of melody, work that is generally more aligned with research in music education takes a more ecological approach to inspecting how musical features affect perception. For example, Long found that length, tonal structure, contour, and individual traits all contribute to performance on melodic dictation examinations and found that structure and tonalness have significant, albeit small predictive powers in modeling (Long 1977). One problem with studies such as Long (1977) is that studies like Long’s make conspicuous methodological decisions such as eliminating individuals from their sample who met an a priori criteria for bad singers. Not only does this reduce the spectrum of ability levels (assuming that singing ability correlates with dictation ability, a finding which has since been established (Norris 2003)), but is additionally flawed in that it is at odds both with the intuition that an individual’s singing ability cannot be taken as a direct representation of their mental image of the melody. In fact, more recent research might suggest that singing ability might instead relate to motor control ability over the vocal tract rather than pitch imagery abilities (Pfordresher and Brown 2007).
Other researchers have also put forward parameters thought to contribute like tempo (Hofstetter 1981), tonality (Dowling 1978; Long 1977; Pembrook 1986; Oura and Hatano 1988), interval motion (Ortmann 1933; Pembrook 1986), length of melody (Long 1977; Pembrook 1986), number of presentations (Hofstetter 1981; Pembrook 1986), context of presentation (Schellenberg and Moore 1985), the background of the listener (Long 1977; Oura and Hatano 1988; Schellenberg and Moore 1985; Taylor and Pembrook 1983) as well as familiarity with a musical style (Schellenberg and Moore 1985). Again we have a listing of studies that consider both structural and experimental aspects of the taxonomy.
Pembrook (1986) provides an extensive detailing of a systematic study to melodic dictation where the authors used tonality, melody length, and type of motion as variables in their experiment. They additionally restricted their experimental melodies to those that were singable. The authors found all three variables to be significant predictors with tonality explaining 13% of the variance, length explaining 3% of the variance and type of motion explaining 1% of the variance. The paper also claimed that people on average can hear and remember 10-16 notes with the quarter note set to 90 beats per minute.
Given the lack of consistent methodologies in administration and scoring of these experiments, it becomes difficult to find ways to generalize basic findings like expected effect sizes– especially when the original materials and data have not been recorded– but there are often interesting theoretical insights to be gleaned. For example, Oura (1991) used a sample of eight people to suggest that when taking melodic dictation, individuals use a system of pattern matching that interfaces with their long term memory in order to complete dictation tasks. While this paper does not bring with it exhaustive evidence supporting this claim, the idea is explored in detail in Chapter 7 when the idea of pattern matching is used in conjunction with Cowan’s Embedded Process model of working memory (Cowan 1988).
More recently, the music education community has also begun to do research around melodic dictation using both qualitative and quantitative methodologies. Paney and Buonviri (2014) interviewed high school teachers on methods they used to teach melodic dictation and among more general findings on teaching methods reported a general awareness and concern among pedagogues regarding the “psychological barriers inherent in learning aural skills”, as well as a general positive disposition to the use of standardized tests used in melodic dictation. Gillespie (2001) surveyed over 40 individual aural skill instructors and reported large discrepancies in how aural skills pedagogues graded and gave feedback on students’ melodic dictations. Other work by Pembrook and Riggins (1990) surveyed various methodologies used by instructors in aural skills settings and reported inconsistencies in grading practice. Some of these studies considered aural skills as a totality like Norris (2003) who provided quantitative evidence to suggest most aural skills pedagogue’s intuition that there is some sort of relationship between melodic dictation and sight singing. Looking at the notorious subset of students with absolute pitch (AP), Dooley and Deutsch (2010) provided empirical evidence that students with AP tend to outperform their non-AP colleagues in tests of dictation.
Continuing to explore the pedagogical literature, Nathan Buonviri and colleagues have also made melodic dictation a central focus of recent work. Using qualitative methods, N. Buonviri (2015) interviewed six sophomore music majors in order to find successful strategies that students engaged in when completing melodic dictations and found evidence to suggest that successful students engage in highly concentrated mental choreography when completing melodic dictations. Nathan O Buonviri and Paney (2015) found that having students sing a preparatory singing pattern after hearing the target melody, essentially a distraction task, hindered performance on melodic dictation. N. Buonviri (2015) found no effects of test presentation format (visual versus aural-visual) using a melodic memory paradigm and more work by Buonviri (2017) reported no significant advantage to listening strategies while partaking in a melodic dictation test.
Not specific to computational musicology or that of the music education literature, other research from music perception has also claimed other experimental features might play a role in dictation. For example, a series of papers by Michael W. Weiss has found a general timbral advantage of voice in memory recall tasks (Weiss et al. 2015; Weiss and Peretz 2019), even finding the effect in amusics. Vocal timbral perception presumably would then have an effect in the recall of music in dictation settings, but evidence supporting other surface features in memory processes has not been as explored (Schellenberg, Stalinski, and Marks 2014).
As documented in this review of the literature on issues that contribute to an individual’s ability to take melodic dictation, the problem is complex. Not only are there difficulties in finding adequate measures of latent psychological constructs assumed to exist and contribute like working memory capacity and musical training, but additionally the amount of musical variables at play that inevitably interact with one another is overwhelming.
Given all the variables that are at play, what then is the best way forward in understanding the processes underlying melodic dictation? In my opinion, the path forward to understanding relies on adopting a polymorphic view of musical abilities for future modeling.
2.4 Polymorphism of Ability
Given the current state of cognitive psychology and psychometrics, as well as advances in computational musicology, the possibilities for now operationalizing and then modeling aspects of melodic dictation are as advanced as they have ever been. The research community can now operationalize every factor that is thought to contribute to this process and has literature to support the recording of almost any variable. This includes concepts from musicianship, to features of a melody, and even unitless measures associated with an individual’s working memory capacity.
While this is certainly possible to do, continuing in this manner of picking variables deemed relevant from such an expansive catalog of parameters will only obfuscate further research. A clearer path forward is needed that reduces the signal to noise ratio in this research. After reviewing this literature, below I list my recommendations for answering this problem.
One of the most important changes to future studies on melodic dictation need avoid the use of latent variables as predictors in statistical models. While abstract concepts like intelligence and musical training are helpful concepts for explaining the variance in responses in aural skills settings, using such abstracted variables describe, but do not explain the causal mechanisms underlying this process.
The most illustrative example of this comes from the above study by Harrison, Asmus, and Serpe (1994) who created a latent variable model of aural skills that was able to predict 74% of the variance in aural skills performance. This latent trait that the authors created is helpful in explaining the patterns of covariance in data, but this would be to reify a statistical abstraction and assume a stance of ontological realsim as noted before (Borsboom, Mellenbergh, and van Heerden 2003). The idea of statistical reification has been critiqued outside of music (Gould 1996; Kovacs and Conway 2016) and additionally has served as the basis for an argument within music (Baker et al. 2018) .
The same arguments put forward in this literature are also relevant in research in aural skills. In order to have a complete, causal model of the processes underlying melodic dictation, it is important to understand melodic dictation as a set of musical abilities that are related to other musical abilities, though may not be unified as a monolithic whole form which individuals draw from in order to execute musical tasks such as melodic dictation. This idea is not new, even in music psychology, as the past two decades have seen calls for a more polymorphic definition of musical ability (Levitin 2012; Peretz and Coltheart 2003) whose modeling will require more concrete ways of defining underlying processes rather than correlating variable together that are helpful at prediction without explaining the process. Using a polymorphic view of musical abilities coupled with a theoretical framework like Karpinski’s will allow for a clearer understanding of the many variables at play during this process.
2.5 Conclusions
In this chapter, I first described what melodic dictation is using Karpinski’s model of melodic dictation. Using his didactic model as a point of departure, I critique what this model does not consider and then put forward a taxonomy of features meant to encompass what this model lacks. I suggested there are both individual as well as musical features that need to be understood in order to have a comprehensive understanding of melodic dictation. Of the two sets of features, individual features can be either cognitive or environmental and musical features can be either structural or experimental. This taxonomy does not consist of exclusive categories and certainly permits interactions between any and all of the levels. Using this taxonomy as a guide, I then surveyed relevant literature in order to discuss how research might effectively quantify each parameter of relevance. Finally, I asserted that in order to provide a more cohesive research program going forward, research on melodic dictation should adopt a polymorphic view of musicianship in line with calls in the past to move away from high level modeling and focus as much as possible on the processes deemed relevant in melodic dictation. The rest of this dissertation will synthesize these areas and put forth novel research contributing to the modeling and subsequent understanding of melodic dictation.
References
Karpinski, Gary. 1990. “A Model for Music Perception and Its Implications in Melodic Dictation.” Journal of Music Theory Pedagogy 4 (1): 191–229.
Karpinski, Gary Steven. 2000. Aural Skills Acquisition: The Development of Listening, Reading, and Performing Skills in College-Level Musicians. Oxford University Press.
Klonoski, Edward. 2006. “Improving Dictation as an Aural-Skills Instructional Tool,” 6.
Potter, Gary. 1990. “Identifying Sucessful Dictation Strategies.” Journal of Music Theory Pedagogy 4 (1): 63–72.
Paney, Andrew S. 2016. “The Effect of Directing Attention on Melodic Dictation Testing.” Psychology of Music 44 (1): 15–24. https://doi.org/10.1177/0305735614547409.
Butler, David. 1997. “Why the Gulf Between Music Perception Research and Aural Training?” Bulletin of the Council for Research in Music Education, no. 132.
Klonoski, Edward. 2000. “A Perceptual Learning Hierarchy: An Imperative for Aural Skills Pedagogy.” College Music Symposium 4: 168–69.
Wright, Colin Richard. 2016. “Investigating Aural: A Case Study of Its Relationship to Degree Success and Its Understanding by University Music Students.” University of Hull.
Baddeley, Alan. 2000. “The Episodic Buffer: A New Component of Working Memory?” Trends in Cognitive Sciences 4 (11): 417–23. https://doi.org/10.1016/S1364-6613(00)01538-2.
Dowling, W. Jay. 1978. “Scale and Contour: Two Components of a Theory of Memory for Melodies.” Psychological Review 84 (4): 341–54.
Dewitt, Lucinda A., and Robert G. Crowder. 1986. “Recognition of Novel Melodies After Brief Delays.” Music Perception: An Interdisciplinary Journal 3 (3): 259–74. https://doi.org/10.2307/40285336.
Oura, Yoko, and Giyoo Hatano. 1988. “Memory for Melodies Among Subjects Differing in Age and Experience in Music.” Psychology of Music 16: 91–109.
Handel, Stephen. 1989. Listening: An Introduction to the Perception of Auditory Events. Cambridge: MIT Press.
Dowling, W. 1990. “Expectancy and Attention in Melody Perception.” Psychomusicology: A Journal of Research in Music Cognition 9 (2): 148–60. https://doi.org/10.1037/h0094150.
Eerola, Tuomas, Jukka Louhivuori, and Edward Lebaka. 2009. “Expectancy in Sami Yoiks Revisited: The Role of Data-Driven and Schema-Driven Knowledge in the Formation of Melodic Expectations.” Musicae Scientiae 13 (2): 231–72. https://doi.org/10.1177/102986490901300203.
Stevens, Catherine J. 2012. “Music Perception and Cognition: A Review of Recent Cross-Cultural Research.” Topics in Cognitive Science 4 (4): 653–67. https://doi.org/10.1111/j.1756-8765.2012.01215.x.
Pearce, Marcus T., and Geraint A. Wiggins. 2012. “Auditory Expectation: The Information Dynamics of Music Perception and Cognition.” Topics in Cognitive Science 4 (4): 625–52. https://doi.org/10.1111/j.1756-8765.2012.01214.x.
Pearce, Marcus T. 2018. “Statistical Learning and Probabilistic Prediction in Music Cognition: Mechanisms of Stylistic Enculturation: Enculturation: Statistical Learning and Prediction.” Annals of the New York Academy of Sciences 1423 (1): 378–95. https://doi.org/10.1111/nyas.13654.
Miller, George A. 1956. “The Magical Number Seven, Plus or Minus Two: Some Limits on Our Capacity for Processing Information.”
Arthur, Claire. 2018. “A Perceptual Study of Scale-Degree Qualia in Context.” Music Perception: An Interdisciplinary Journal 35 (3): 295–314. https://doi.org/10.1525/mp.2018.35.3.295.
Taylor, Jack A., and Randall G. Pembrook. 1983. “Strategies in Memory for Short Melodies: An Extension of Otto Ortmann’s 1933 Study.” Psychomusicology: A Journal of Research in Music Cognition 3 (1): 16–35. https://doi.org/10.1037/h0094258.
Goldman, Andrew, Tyreek Jackson, and Paul Sajda. 2018. “Improvisation Experience Predicts How Musicians Categorize Musical Structures.” Psychology of Music, June, 030573561877944. https://doi.org/10.1177/0305735618779444.
Lane, David M., and Yu-Hsuan A. Chang. 2018. “Chess Knowledge Predicts Chess Memory Even After Controlling for Chess Experience: Evidence for the Role of High-Level Processes.” Memory & Cognition 46 (3): 337–48. https://doi.org/10.3758/s13421-017-0768-2.
Meyer, Leonard. 1956. Emotion and Meaning in Music. Chicago: University of Chicago Press.
Ritchie, Stuart. 2015. Intelligence: All That Matters. All That Matters. Hodder & Stoughton.
Unsworth, Nash, Richard P. Heitz, Josef C. Schrock, and Randall W. Engle. 2005. “An Automated Version of the Operation Span Task.” Behavior Research Methods 37 (3): 498–505. https://doi.org/10.3758/BF03192720.
Gould, Stephan Jay. 1996. The Mismeasure of Man. WW Norton & Company.
Darwin, Charles. 1859. On the Origin of Species. Routledge.
Spearman, Charles. 1904. “"General Intelligence," Objectively Determined and Measured,” 93.
Cattell, R. 1971. Abilities: Their Growth, Structure, and Action. Boston, MA: Houghton Mifflin.
J Horn. 1994. “Theory of Fluid and Crystalized Intelligence.” In Encyclopedia of Human Intelligence, 443–51. R. Sternberg. New York: MacMillan Reference Library.
Matzke, Dora, Conor V. Dolan, and Dylan Molenaar. 2010. “The Issue of Power in the Identification of ‘G’ with Lower-Order Factors.” Intelligence 38 (3): 336–44. https://doi.org/10.1016/j.intell.2010.02.001.
Kovacs, Kristof, and Andrew R. A. Conway. 2016. “Process Overlap Theory: A Unified Account of the General Factor of Intelligence.” Psychological Inquiry 27 (3): 151–77. https://doi.org/10.1080/1047840X.2016.1153946.
Borsboom, Denny, Gideon J. Mellenbergh, and Jaap van Heerden. 2003. “The Theoretical Status of Latent Variables.” Psychological Review 110 (2): 203–19. https://doi.org/10.1037/0033-295X.110.2.203.
Cowan, Nelson. 2005. Working Memory Capacity. Working Memory Capacity. New York, NY, US: Psychology Press. https://doi.org/10.4324/9780203342398.
Broadbent, Donald E (Donald Eric). 1958. Perception and Communication. Pergamon.
Atkinson, R C, and R M Shiffrin. 1968. “HUMAN MEMORY: A PROPOSED SYSTEM AND ITS CONTROL PROCESSES!” Human Memory, 54.
Baddeley, Alan D., and Graham Hitch. 1974. “Working Memory.” In Psychology of Learning and Motivation, 8:47–89. Elsevier. https://doi.org/10.1016/S0079-7421(08)60452-1.
Berz, William L. 1995. “Working Memory in Music: A Theoretical Model.” Music Perception: An Interdisciplinary Journal 12 (3): 353–64. https://doi.org/10.2307/40286188.
Williamson, Victoria J., Alan D. Baddeley, and Gramham J. Hitch. 2010. “Musicians’ and Nonmusicians’ Short-Term Memory for Verbal and Musical Sequences: Comparing Phonological Similarity and Pitch Proximity.” Memory & Cognition 38 (2): 163–75. https://doi.org/10.3758/MC.38.2.163.
Wöllner, Clemens, and Andrea R. Halpern. 2016. “Attentional Flexibility and Memory Capacity in Conductors and Pianists.” Attention, Perception, & Psychophysics 78 (1): 198–208. https://doi.org/10.3758/s13414-015-0989-z.
Cowan, Nelson. 1988. “Evolving Conceptions of Memory Storage, Selective Attention, and Their Mutual Constraints Within the Human Information-Processing System.” Psychological Bulletin 104 (2): 163–91.
Miller, George A. 1989. A History of Psychology in Autobiography. L. Gardner. Vol. VIII. Stanford, CA: Stanford University Press.
Cowan, Nelson. 2010. “The Magical Mystery Four: How Is Working Memory Capacity Limited, and Why?” Current Directions in Psychological Science 19 (1): 51–57. https://doi.org/10.1177/0963721409359277.
Unsworth, Nash, Thomas S. Redick, Richard P. Heitz, James M. Broadway, and Randall W. Engle. 2009. “Complex Working Memory Span Tasks and Higher-Order Cognition: A Latent-Variable Analysis of the Relationship Between Processing and Storage.” Memory 17 (6): 635–54. https://doi.org/10.1080/09658210902998047.
Conway, Andrew R. A., Michael J. Kane, Michael F. Bunting, D. Zach Hambrick, Oliver Wilhelm, and Randall W. Engle. 2005. “Working Memory Span Tasks: A Methodological Review and User’s Guide.” Psychonomic Bulletin & Review 12 (5): 769–86. https://doi.org/10.3758/BF03196772.
Krumhansl, Carol. 2001. Cognitive Foundations of Musical Pitch. Oxford University Press.
Schenker, Heinrich. 1935. Der Freie Satz. Vol. III. Universal Edition.
Saffran, Jenny R, Elizabeth K Johnson, Richard N Aslin, and Elissa L Newport. 1999. “Statistical Learning of Tone Sequences by Human Infants and Adults.” Cognition 70 (1): 27–52. https://doi.org/10.1016/S0010-0277(98)00075-4.
Talamini, Francesca, Gianmarco Altoè, Barbara Carretti, and Massimo Grassi. 2017. “Musicians Have Better Memory Than Nonmusicians: A Meta-Analysis.” Edited by Lutz Jäncke. PLOS ONE 12 (10): e0186773. https://doi.org/10.1371/journal.pone.0186773.
Kopiez, Reinhard, and Ji Lee. 2006. “Towards a Dynamic Model of Skills Involved in Sight Reading Music.” Music Education Research 8 (1): 97–120. https://doi.org/10.1080/14613800600570785.
Kopiez, Reinhard, and Ji Lee. 2008. “Towards a General Model of Skills Involved in Sight Reading Music.” Music Education Research 10 (1): 41–62. https://doi.org/10.1080/14613800701871363.
Meinz, Elizabeth J., and David Z. Hambrick. 2010. “Deliberate Practice Is Necessary but Not Sufficient to Explain Individual Differences in Piano Sight-Reading Skill: The Role of Working Memory Capacity.” Psychological Science 21 (7): 914–19. https://doi.org/10.1177/0956797610373933.
Nichols, Bryan E., Clemens Wöllner, and Andrea R. Halpern. 2018. “Score One for Jazz: Working Memory in Jazz and Classical Musicians.” Psychomusicology: Music, Mind, and Brain 28 (2): 101–7. https://doi.org/10.1037/pmu0000211.
Engle, Randall W. 2002. “Working Memory Capacity as Executive Attention.” Current Directions in Psychological Science 11 (1): 19–23. https://doi.org/10.1111/1467-8721.00160.
Colley, Ian D, Peter E Keller, and Andrea R Halpern. 2017. “Working Memory and Auditory Imagery Predict Sensorimotor Synchronisation with Expressively Timed Music.” Quarterly Journal of Experimental Psychology 71 (8): 1781–96. https://doi.org/10.1080/17470218.2017.1366531.
Müllensiefen, Daniel, Peter Harrison, Francesco Caprini, and Amy Fancourt. 2015. “Investigating the Importance of Self-Theories of Intelligence and Musicality for Students’ Academic and Musical Achievement.” Frontiers in Psychology 6 (November). https://doi.org/10.3389/fpsyg.2015.01702.
Schellenberg, E Glenn. 2017. “Music and Nonmusical Abilities,” 17.
Gibson, Crystal, Bradley S. Folley, and Sohee Park. 2009. “Enhanced Divergent Thinking and Creativity in Musicians: A Behavioral and Near-Infrared Spectroscopy Study.” Brain and Cognition 69 (1): 162–69. https://doi.org/10.1016/j.bandc.2008.07.009.
Hille, Katrin, Kilian Gust, Ulrich Bitz, and Thomas Kammer. 2011. “Associations Between Music Education, Intelligence, and Spelling Ability in Elementary School.” Advances in Cognitive Psychology 7 (-1): 1–6. https://doi.org/10.2478/v10053-008-0082-4.
Schellenberg, E. 2011. “Examining the Association Between Music Lessons and Intelligence: Music Lessons and Intelligence.” British Journal of Psychology 102 (3): 283–302. https://doi.org/10.1111/j.2044-8295.2010.02000.x.
Schellenberg, E. Glenn, and Monika Mankarious. 2012. “Music Training and Emotion Comprehension in Childhood.” Emotion 12 (5): 887–91. https://doi.org/10.1037/a0027971.
Corrigall, Kathleen A., and E. Glenn Schellenberg. 2015. “Predicting Who Takes Music Lessons: Parent and Child Characteristics.” Frontiers in Psychology 6 (March). https://doi.org/10.3389/fpsyg.2015.00282.
Degé, Franziska, Claudia Kubicek, and Gudrun Schwarzer. 2011. “Music Lessons and Intelligence: A Relation Mediated by Executive Functions.” Music Perception: An Interdisciplinary Journal 29 (2): 195–201. https://doi.org/10.1525/mp.2011.29.2.195.
Schellenberg, E. 2006. “Long-Term Positive Associations Between Music Lessons and IQ.” Journal of Educational Psychology 98 (2): 457–68. https://doi.org/10.1037/0022-0663.98.2.457.
Corrigall, Kathleen A., and Laurel J. Trainor. 2011. “Associations Between Length of Music Training and Reading Skills in Children.” Music Perception: An Interdisciplinary Journal 29 (2): 147–55. https://doi.org/10.1525/mp.2011.29.2.147.
Parbery-Clark, Alexandra, Dana L. Strait, Samira Anderson, Emily Hittner, and Nina Kraus. 2011. “Musical Experience and the Aging Auditory System: Implications for Cognitive Abilities and Hearing Speech in Noise.” Edited by Peter Csermely. PLoS ONE 6 (5): e18082. https://doi.org/10.1371/journal.pone.0018082.
Strait, Dana L., Alexandra Parbery-Clark, Emily Hittner, and Nina Kraus. 2012. “Musical Training During Early Childhood Enhances the Neural Encoding of Speech in Noise.” Brain and Language 123 (3): 191–201. https://doi.org/10.1016/j.bandl.2012.09.001.
Francois, C., J. Chobert, M. Besson, and D. Schon. 2013. “Music Training for the Development of Speech Segmentation.” Cerebral Cortex 23 (9): 2038–43. https://doi.org/10.1093/cercor/bhs180.
Moreno, Sylvain, Carlos Marques, Andreia Santos, Manuela Santos, São Luís Castro, and Mireille Besson. 2009. “Musical Training Influences Linguistic Abilities in 8-Year-Old Children: More Evidence for Brain Plasticity.” Cerebral Cortex 19 (3): 712–23. https://doi.org/10.1093/cercor/bhn120.
Mehr, Samuel A., Adena Schachner, Rachel C. Katz, and Elizabeth S. Spelke. 2013. “Two Randomized Trials Provide No Consistent Evidence for Nonmusical Cognitive Benefits of Brief Preschool Music Enrichment.” Edited by Marina Pavlova. PLoS ONE 8 (12): e82007. https://doi.org/10.1371/journal.pone.0082007.
Swaminathan, Swathi, E. Glenn Schellenberg, and Safia Khalil. 2017. “Revisiting the Association Between Music Lessons and Intelligence: Training Effects or Music Aptitude?” Intelligence 62 (May): 119–24. https://doi.org/10.1016/j.intell.2017.03.005.
Ericsson, K Anders, Ralf Th Krampe, and Clemens Tesch-Romer. 1993. “The Role of Deliberate Practice in the Acquisition of Expert Performance.”
Detterman, Douglas K., and Joanne Ruthsatz. 1999. “Toward a More Comprehensive Theory of Exceptional Abilities.” Journal for the Education of the Gifted 22 (2): 148–58. https://doi.org/10.1177/016235329902200204.
Ruthsatz, Joanne, Douglas Detterman, William S. Griscom, and Britney A. Cirullo. 2008. “Becoming an Expert in the Musical Domain: It Takes More Than Just Practice.” Intelligence 36 (4): 330–38. https://doi.org/10.1016/j.intell.2007.08.003.
Mosing, Miriam A., Guy Madison, Nancy L. Pedersen, Ralf Kuja-Halkola, and Fredrik Ullén. 2014. “Practice Does Not Make Perfect: No Causal Effect of Music Practice on Music Ability.” Psychological Science 25 (9): 1795–1803. https://doi.org/10.1177/0956797614541990.
Baker, David, Juan Ventura, Matthew Calamia, Daniel Shanahan, and Emily Elliott. 2018. “Examining Musical Sophistication: A Replication and Theoretical Commentary on the Goldsmiths Musical Sophistication Index.” Musicae Scientiae.
Blacking, John. 2000. How Musical Is Man? 6. printing. The Jessie and John Danz Lectures. Seattle: University of Washington Press.
Murphy, Christopher. 1999. “How Far Do Tests of Musical Ability Shed Light on the Nature of Musical Intelligence?” British Journal of Music Education 16 (1): 39–50. https://doi.org/10.1017/S0265051799000133.
Chenette, Timothy K. 2019. “Reframing Aural Skills Instruction Based on Research in Working Memory.” Journal for Music Theory Pedagogy, 18.
Furby, Victoria J. 2016. “The Effects of Peer Tutoring on the Aural Skills Performance of Undergraduate Music Majors.” Update: Applications of Research in Music Education 34 (3): 33–39. https://doi.org/10.1177/8755123314556547.
Harrison, Carole S., Edward P. Asmus, and Richard T. Serpe. 1994. “Effects of Musical Aptitude, Academic Ability, Music Experience, and Motivation on Aural Skills.” Journal of Research in Music Education 42 (2): 131. https://doi.org/10.2307/3345497.
Banton, Louise. 1995. “The Role of Visual and Auditory Feedback During Sight-Reading of Music.” Psychology of Music 23 (1): 3–16.
Bland, Leland. 1984. Sight Singing Through Melodic Analysis: A Guide to the Study of Sight Singing and an Aid to Ear Training Instruction. Scarecrow Press.
Root, Frederick. 1931. Methodical Sight-Singing: Lessons in Vocal Culture. Bryn Mawr, Pennsylvania: Theodore Presser Co.
Fournier, Guillaume, Maria Teresa Moreno Sala, Francis Dubé, and Susan O’Neill. 2017. “Cognitive Strategies in Sight-Singing: The Development of an Inventory for Aural Skills Pedagogy.” Psychology of Music, December, 030573561774514. https://doi.org/10.1177/0305735617745149.
Asmus, Edward P. 2004. “Music Teaching and Music Literacy.” Journal of Music Teacher Education 13 (2): 6–8. https://doi.org/10.1177/10570837040130020102.
Thompson, Kathy. 2003. “Pitch Internalization Strategies of Professional Musicians.” PhD thesis, University of Oklahoma.
Rogers, Michael R. 2004. Teaching Approaches in Music Theory: An Overview of Pedagogical Philosophies. 2nd ed. Carbondale: Southern Illinois University Press.
Harrison, Carole. 1987. “The Validity and Musical Aptitude Profile for Predicting Grades in Freshman Music Theory.” Educational and Psychological Measurment 47 (2): 477–82.
Cambouropoulos, Emilios. 2009. “How Similar Is Similar?” Musicae Scientiae 13 (1_suppl): 7–24. https://doi.org/10.1177/102986490901300102.
Wiggins, Geraint A. 2007. “Models of Musical Similarity.” Musicae Scientiae 11 (1_suppl): 315–38. https://doi.org/10.1177/102986490701100112.
Clarke, Eric F. 2005. Ways of Listening: An Ecological Approach to the Perception of Musical Meaning. Oxford: Oxford Univ. Pr.
Salzer, Felix, and Leopold Mannes. 1982. Structural Hearing: Tonal Coherence in Music. New York: Dover.
Schachter, Carl. 2006. Schenker Studies 2. Cambridge: Cambridge University Press.
Schenker, Heinrich, Hedi Siegel, and Carl Schachter, eds. 1990. Schenker Studies. Cambridge ; New York: Cambridge University Press.
Agawu, Kofi. 2004. “How We Got Out of Analysis, and How to Get Back in Again.” Music Analysis 23 (2-3): 267–86. https://doi.org/10.1111/j.0262-5245.2004.00204.x.
Kerman, Joseph. 1986. Contemplating Music: Challenges to Musicology. Cambridge, Mass: Harvard Univ. Press.
Narmour, Eugene. 1990. The Analysis and Cognition of Basic Melodic Structures: The Implication-Realization Model. Chicago: University of Chicago Press.
Narmour, Eugene. 1992. The Analysis and Cognition of Melodic Complexity: The Implication-Realization Model. University of Chicago Press.
Schellenberg, E. Glenn. 1997. “Simplifying the Implication-Realization Model of Melodic Expectancy.” Music Perception: An Interdisciplinary Journal 14 (3): 295–318. https://doi.org/10.2307/40285723.
Margulis, Elizabeth Hellmuth. 2005. “A Model of Melodic Expectation.” Music Perception: An Interdisciplinary Journal 22 (4): 663–714. https://doi.org/10.1525/mp.2005.22.4.663.
Huron, David. 2006. Sweet Anticipation. MIT Press.
Pearce, Marcus. 2005. “The Construction and Evaluation of Statistical Models of Melodic Structure in Music Perception and Composition.” PhD thesis, Department of Computer Science: City University of London.
Conklin, Darrell, and Ian H. Witten. 1995. “Multiple Viewpoint Systems for Music Prediction.” Journal of New Music Research 24 (1): 51–73. https://doi.org/10.1080/09298219508570672.
Ortmann, Otto. 1933. “Some Tonal Determinants of Melodic Memory.” Journal of Educational Psychology 24 (6): 454–67. https://doi.org/10.1037/h0075218.
Huron, David. 1994. “The Humdrum Toolkit: Reference Manual.” Center for Computer Assisted Research in the Humanities.
Cuthbert, Michael Scott, and Christopher Ariza. 2010. “Music21: A Toolkit for Computer-Aided Musicology and Symbolic Music Data,” 7.
Huron, David. 1996. “The Melodic Arch in Western Folk Songs.” Computing in Musicology 10: 3–23.
Schaffrath, Helmuth. 1995. “The Essen Folk Song Collection, D. Huron.”
Grabe, Esther. 2002. “Durational Variability in Speech and the Rhythm Class Hypothesis.” Papers in Laboratory Phonology, 16.
Albrecht, Joshua, and Daniel Shanahan. 2013. “The Use of Large Corpora to Train a New Type of Key-Finding Algorithm: An Improved Treatment of the Minor Mode.” Music Perception: An Interdisciplinary Journal 31 (1): 59–67. https://doi.org/10.1525/mp.2013.31.1.59.
Mullensiefen, Daniel. 2009. “Fantastic: Feature ANalysis Technology Accessing STatistics (in a Corpus): Technical Report V1.5.”
Manning, Christopher D., and Hinrich Schütze. 1999. Foundations of Statistical Natural Language Processing. Cambridge, Mass: MIT Press.
Müllensiefen, Daniel, and Marc Pendzich. 2009. “Court Decisions on Music Plagiarism and the Predictive Value of Similarity Algorithms.” Musicae Scientiae 48: 257–95.
Kopiez, Reinhard, and Daniel Mullensiefen. 2011. “Auf Der Suche Nach Den ‘Popularitätsfaktoren’ in Den Song-Melodien Des Beatles-Albums Revolver: Eine Computergestützte Feature-Analyse [in Search of Features Explaining the Popularity of the Tunes from the Beatles Album Revolver: A Computer-Assisted Feature Analysis].” Musik Und Popularitat. Beitrage Zu Einer Kulturgeschichte Zwischen, 207–25.
Müllensiefen, Daniel, and Andrea R. Halpern. 2014. “The Role of Features and Context in Recognition of Novel Melodies.” Music Perception: An Interdisciplinary Journal 31 (5): 418–35. https://doi.org/10.1525/mp.2014.31.5.418.
Jakubowski, Kelly, Sebastian Finkel, Lauren Stewart, and Daniel Müllensiefen. 2017. “Dissecting an Earworm: Melodic Features and Song Popularity Predict Involuntary Musical Imagery.” Journal of Aesthetics, Creativity, and the Arts 11 (2): 112–35.
Williamson, Victoria J, and Daniel Müllensiefen. 2012. “Earworms from Three Angles: Situational Antecedents, Personality Predisposition and the Quest for a Musical Formula.” Proceedings from the 12th International Conference on Music Perception and Cognition, 1124–32.
Balen, Jan Van, John Ashley Burgoyne, and Dimitrios Bountouridis. 2015. “Corpus Analyis Tools for Computational Hook Discovery.” Proceedings of International Society for Music Information Retrevial 15.
Baker, David J., and Daniel Müllensiefen. 2017. “Perception of Leitmotives in Richard Wagner’s Der Ring Des Nibelungen.” Frontiers in Psychology 8 (May). https://doi.org/10.3389/fpsyg.2017.00662.
Harrison, Peter M.C., Jason Jiří Musil, and Daniel Müllensiefen. 2016. “Modelling Melodic Discrimination Tests: Descriptive and Explanatory Approaches.” Journal of New Music Research 45 (3): 265–80. https://doi.org/10.1080/09298215.2016.1197953.
Rainsford, M., M. A. Palmer, and G. Paine. 2018. “The MUSOS (MUsic SOftware System) Toolkit: A Computer-Based, Open Source Application for Testing Memory for Melodies.” Behavior Research Methods 50 (2): 684–702. https://doi.org/10.3758/s13428-017-0894-6.
Shannon, Claude. 1948. “A Mathematical Theory of Communication.” Bell System Technical Journal 27: 379–423.
Sauve, Sarah. 2017. “Prediction in Polyphony: Modelling Auditory Scene Analysis.” PhD thesis, Centre for Digital Music: Queen Mary, University of London.
Harrison, Peter M . C., and Marcus Thomas Pearce. 2018. “Dissociating Sensory and Cognitive Theories of Harmony Perception Through Computational Modeling.” https://doi.org/10.31234/osf.io/wgjyv.
Perruchet, Pierre, and Sebastien Pacton. 2006. “Implicit Learning and Statistical Learning: One Phenomenon, Two Approaches.” Trends in Cognitive Sciences 10 (5): 233–38. https://doi.org/10.1016/j.tics.2006.03.006.
Jamieson, Randall K., and D. J. K. Mewhort. 2009. “Applying an Exemplar Model to the Serial Reaction-Time Task: Anticipating from Experience.” Quarterly Journal of Experimental Psychology 62 (9): 1757–83. https://doi.org/10.1080/17470210802557637.
Long, Peggy A. 1977. “Relationships Between Pitch Memory in Short Melodies and Selected Factors.” Journal of Research in Music Education 25 (4): 272–82. https://doi.org/10.2307/3345268.
Norris, Charles. 2003. “The Relationship Between Sight Singing Achievement and Melodic Dictation Achievement.” Contributions to Music Education 30 (1): 39–53.
Pfordresher, Peter Q., and Steven Brown. 2007. “Poor-Pitch Singing in the Absence of "Tone Deafness".” Music Perception: An Interdisciplinary Journal 25 (2): 95–115. https://doi.org/10.1525/mp.2007.25.2.95.
Hofstetter, Fred T. 1981. “Computer-Baesed Recognition of Perceptual Patterns and Learning Styles in Rhythmic Dictation Exercises.” Journal of Research in Music Education 29 (4): 265–77. https://doi.org/10.2307/3345003.
Pembrook, Randall G. 1986. “Interference of the Transcription Process and Other Selected Variables on Perception and Memory During Melodic Dictation.” Journal of Research in Music Education 34 (4): 238. https://doi.org/10.2307/3345259.
Schellenberg, Stephen, and Randall S. Moore. 1985. “The Effect of Tonal-Rhythmic Context on Short-Term Memory of Rhythmic and Melodic Sequences.” Bulletin of the Council for Research in Music Education, no. 85: 207–17.
Oura, Yoko. 1991. “Constructing a Representation of a Melody: Transforming Melodic Segments into Reduced Pitch Patterns Operated on by Modifiers.” Music Perception: An Interdisciplinary Journal 9 (2): 251–65. https://doi.org/10.2307/40285531.
Paney, Andrew S., and Nathan O. Buonviri. 2014. “Teaching Melodic Dictation in Advanced Placement Music Theory.” Journal of Research in Music Education 61 (4): 396–414. https://doi.org/10.1177/0022429413508411.
Gillespie, Jeffrey L. 2001. “Melodic Dictation Scoring Methods: An Exploratory Study.” Journal for Music Theory Pedagogy 15.
Pembrook, Randall G., and H. Lee Riggins. 1990. “"Send Help!": Aural Skills Instruction in U.S. Colleges and Universities.” Journal of Music Theory Pedagogy 4 (1): 230–41.
Dooley, Kevin, and Diana Deutsch. 2010. “Absolute Pitch Correlates with High Performance on Musical Dictation.” The Journal of the Acoustical Society of America 128 (2): 890–93. https://doi.org/10.1121/1.3458848.
Buonviri, Nathan. 2015. “Effects of Music Notation Reinforcement on Aural Memory for Melodies.” International Journal of Music Education 33 (4): 442–50. https://doi.org/10.1177/0255761415582345.
Buonviri, Nathan O, and Andrew S Paney. 2015. “Melodic Dictation Instruction.” Journal of Research in Music Education 62 (2): 224–37.
Buonviri, Nathan O. 2017. “Effects of Two Listening Strategies for Melodic Dictation.” Journal of Research in Music Education 65 (3): 347–59. https://doi.org/10.1177/0022429417728925.
Weiss, Michael W., Patrícia Vanzella, E. Glenn Schellenberg, and Sandra E. Trehub. 2015. “Rapid Communication: Pianists Exhibit Enhanced Memory for Vocal Melodies but Not Piano Melodies.” Quarterly Journal of Experimental Psychology 68 (5): 866–77. https://doi.org/10.1080/17470218.2015.1020818.
Weiss, Michael W., and Isabelle Peretz. 2019. “Ability to Process Musical Pitch Is Unrelated to the Memory Advantage for Vocal Music.” Brain and Cognition 129 (February): 35–39. https://doi.org/10.1016/j.bandc.2018.11.011.
Schellenberg, E. Glenn, Stephanie M. Stalinski, and Bradley M. Marks. 2014. “Memory for Surface Features of Unfamiliar Melodies: Independent Effects of Changes in Pitch and Tempo.” Psychological Research 78 (1): 84–95. https://doi.org/10.1007/s00426-013-0483-y.
Levitin, Daniel J. 2012. “What Does It Mean to Be Musical?” Neuron 73 (4): 633–37. https://doi.org/10.1016/j.neuron.2012.01.017.
Peretz, Isabelle, and Max Coltheart. 2003. “Modularity of Music Processing.” Nature Neuroscience 6 (7): 688–91. https://doi.org/10.1038/nn1083.
In his highly influential book Aural Skills Acquisition: The Development of Listening, Reading, and Performing Skills in College-Level Musicians, Karpinski (2000) documents this sentiment in music pedagogy circles by highlighting poetic adages from Romantic composer Robert Schumann in the mid-19th century through 21st century music educator Charles Elliott, thus providing examples of the belief that improving one’s aural skills, or ear, is a highly sought after skill.↩
And in his Figure 3.1 he does caption it as an idealized dictation process↩
HOW DO I CITE THIS BC ITS HAPPENING AT CONFERNCES BUT NOT PUBLISHED AND I DONT WANT THE TEXT DATED↩
Gould puts forward a complete, yet very charged reading of the early history of cognitive testing and his writings on the subject have been accused of falling prey to the same logic he rails against (Warne et al. 2019)↩